The advent of ChatGPT and its many competitors certainly puts younger workers in mind of automation and its effect on their careers in the near future. In 2023, it’s unclear to most people under 40 how automation is going to affect their jobs going forward.
Today we’re going to give you a brief overview of the most important short and medium term effects of automation on the workplace, and the jobs it’s most likely, as well as least likely to affect. While the truth is that most jobs are going to be deeply affected by continued improvements in computerized automation long term, there are certainly careers and jobs that will experience the effects far more quickly than others.
The TL;DR is that although automation is likely to change the nature of jobs in the future, it may not have as deep an impact on the jobs most people see as “at risk” from automation. Instead, advances in machine learning are more likely to greatly change the types of jobs that are often seen as “safe” from automation, partly because it is these jobs that deal with the most volume of complex information, but also because it is these jobs where the greatest “cost savings” can be achieved from automation.
Automation: Not About McJobs
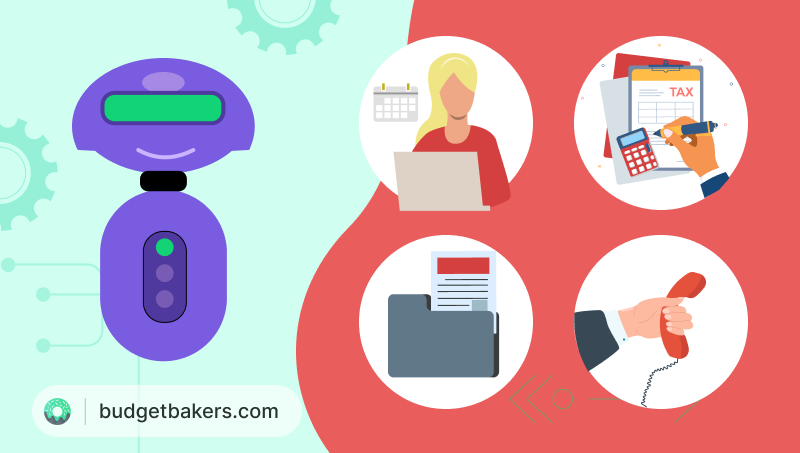
When automation is described in the media, it is most often represented by robotics: the automation of physical processes that are currently done by humans, or by machines that are currently controlled by humans.
We’re taught to view automation as the elimination of some manual labor task which is repetitive and potentially dangerous. While these types of jobs are certainly always at risk of being automated, the truth is that robotic automation has already had its deepest impacts on manufacturing. Today, manufacturing, mining, construction, and other heavy industrial jobs are already heavily automated. The automation takes the form of heavy machinery, 3d printers, presses, etc.
The jobs that still exist in these industries are typically those that are absolutely required for reasons of safety and oversight. After all, when most jobs in an assembly line have been handed over to machines, the few human jobs left become far harder to automate, as they require a broader knowledge of the operations of a factory, and must also replace humans as a key point of oversight and safety. It is not turning a wrench or swinging a hammer, but rather overseeing an entire line, or fixing a machine that requires a human touch.
The same is actually true of what we often consider to be “low skill” jobs adjacent to manufacturing, such as restaurant service. The truth is that a restaurant chain like McDonalds is already heavily automated, with most of the labor having been replaced by machines years ago, with food and ingredients produced on an industrial scale. Large and efficient food production plants do most of the work of producing food, and workers on the restaurant side are typically doing fairly complicated quality control and maintenance tasks that a machine can’t be left unattended to do.
The few workers who remain in food service are often vital to providing the small amount of manual labor that cannot be effectively automated, such as quality control, customer service, and security. So work is not disappearing in these areas of the economy. Instead, a different technology is being developed: it takes two important forms: RPA (Robotic Process Automation) and ML (Machine Learning).
What Real Automation Looks Like Today

Robotic Process Automation (RPA) is easiest to understand by comparing it to the work of physical robots. RPA takes repetitive non-physical tasks that humans have been doing, and assigns this process to a computer, or even occasionally to a physical robot. Thus such routine activities as transcribing data, checking account sums, and verifying identities are now done by RPA systems. Instead of humans receiving information from one system and putting it into another (such as a bank employee receiving a cheque and entering it into a bank’s account software), these tasks are increasingly done by machines.
RPA allows the work of originally disconnected computer systems to be coordinated, so that one system that is not designed to talk to other systems can do so efficiently. RPA thus eliminates many tasks which were done by skilled professionals only a few years ago. It does not necessarily eliminate a skilled professional job, but it strongly affects how professionals spend much of their time working. RPA frequently eliminates the most time-wasting activities that information workers endure, such as reading data, sending information via email, or verifying customer information.
In the coming years, those aspects of daily work that involve these kinds of repetitive tasks will become more automated. If you currently spend a lot of time moving information from one computer system to another, expect that this aspect of your work will be increasingly automated.
Machine Learning (ML) is a related technology that uses a large amount of data to “teach” computers how to perform sometimes complex tasks by showing them countless examples of the work being done. These systems are often supported by data input professionals who work to help train the algorithms to get the right answers most of the time. For example, the software that now reads your handwriting on postal envelopes involves machine learning, and there is actually a team of handwriting experts who sit in an office somewhere in your country, reviewing any example of handwriting that the machine cannot comprehend with a high degree of certainty.
Machine learning, while it is often referred to as a kind of “artificial intelligence,” is in fact more like a probability engine. It builds a probabilistic model of a series of decisions based on extremely basic pieces of information, and makes decisions based on that model. Occasionally, and ever more rarely, an ML system requires a human to check its work. Yet systems that control important informational tasks like payment orders and checks now operate with a higher degree of accuracy and confidence than a trained human can achieve at the same job. And obviously, the machines can do this kind of work infinitely faster than a human, for a relatively tiny fraction of the cost.
In the coming years, any work that requires you to make a series of small but important decisions or inferences will be affected by machine learning. The ability to recognize handwriting, coordinate a series of images, or recognize items in a photograph, for example, defines tasks that machine learning will be used for.
ML and RPA are “Invisible Automation”
RPA and ML technologies have fewer effects on the physical world than the kinds of automation that we’ve been used to for decades. But why?
It’s simple to understand if you consider how economies today are continuing to grow. Whereas in the past, developed economies had plenty of room to grow in terms of their material wealth, producing more products like fuel, food, cars, machines, consumer products, and other comforts, today the main growth product in modern society is data.
As the amount and types of data that are being produced, collected, and used increases, the opportunities for RPA and ML to impact society also increase. The more you use online services, for example, the more data you produce. And the more data you produce, the more opportunities companies have to influence your decisions, or use those decisions to create valuable services for you.
In a pre “big data” economy, the primary means of increasing profitability was to influence someone’s decision making at the point of purchase for specific products. Companies competed over selling a consumer one particular product, based on a set of features and a price. Today, increasingly, consumer products are indistinguishable from each other, and instead these companies compete over providing ongoing services, such as content subscriptions, and software.
Whereas in 1980, you would have made the “important” economic decision of buying a new TV, based on what features the different products offered, today the “important” economic decision is which content service you subscribe to, or which you choose to cancel. Therefore the TV you buy is less important, and therefore the differentiation between these products is less critical, economically. Therefore many of these products are now virtually identical, with an identical set of features. Companies no longer make most of their money when you buy a product in the store, so the need for these products to be different is disappearing.
As the amount of data we continue to produce only grows, the impact of this kind of automation will grow. As the availability of biotelemetric data, DNA, and eventually perhaps even brain activity data increases, along with other complex data sets like those of weather and traffic systems, the more ML and RPA will be used to put that data to an effective use on behalf of the consumer of electronic services.
All of that may sound dystopian, and maybe it is, but it also teaches us an important lesson: automation follows the part of the economy that is growing. As the population’s growth slows, manufacturing, mining, heavy industry, energy, and transportation slow in their growth as well. When growth is slowing, automation makes less and less economic sense. It no longer makes much sense, economically, to innovate new physical features for consumer products. Now it makes more sense to innovate around software capabilities that a consumer will pay for over and over again. Therefore this is where we can expect the most change to occur.
As the importance of data grows, the importance of physical automation decreases. Despite the advances in robotics technology, we might not expect that physical automation will have such a significant impact on the future, compared to the impact it has had as our population has grown rapidly over the last few centuries. Today, we produce orders of magnitude more data every year, but we do not buy orders of magnitude more physical products.
It’s all About Data
As we’ve seen, automation is continuing to increase, but it is increasing in correlation with the amount of data we produce. We should look at jobs connected with the growth of data to understand the true impacts of automation in the near future.
What this suggests is that the jobs most impacted by automation in the near future will be those that require the most data. Medicine, genomics, natural sciences, pharmacology, advertising, software engineering, design, and other areas involving hard science are likely to see the deepest impact from automation in the next few decades. This is simply because these jobs involve the most data, and the fastest increase in the overall availability of data that requires automation to be used.
Jobs that are either disconnected from, or somehow not impacted by the amount of data available will probably experience the least impact from automation. This may mean that jobs such as teaching, customer service and sales, nursing, maintenance and repair, and creative work will see some of the fewest impacts. Yet even here, the impacts will not be minimal, or even small. These jobs will still be heavily influenced by the availability of new data technologies and methods, but the essence of this kind of work will remain mostly the same. No amount of data automation can repair a punctured tire, or bring a hamburger to a table. Though these jobs may seem simple, they are resistant to automation simply because they do so many different things so efficiently already.
Types of Work Most Affected by Automation

As we’ve established, the jobs that are most likely to be directly effected by automation (which is not to say the jobs that are at risk from automation), are jobs that are strongly dependent on the amount of data one uses.
This means professions in diagnostic medicine, material science, earth science, energy, climate related work, digital arts, and software engineering are most likely to substantially change thanks to the increasing availability of data. But as we’ve seen, those jobs are not necessarily going to be eliminated because of automation. Just because an artist can do a lot more thanks to AI doesn’t mean the artist loses their job. However, an artist that formerly needed 3 or 4 collaborators may now not need them. Certainly this is most true for the professions that surround that industry, and support it without directly engaging with the production of art, or scientific research, or medicine, or energy.
Jobs directly at risk from automation are those that are seeing a large increase in the amount of data available, and where the work being done is relatively routine. These are seen as more “administrative” jobs, that are not specifically management roles. Thus, accounting, regulatory and financial oversight, legal work, HR, communications specialists, and other support workers may find fewer opportunities because much of their work is being automated.
Of course, people in these professions have also been facing pressure from automation for years. Younger workers will not remember a time in which middle managers at corporations all had their own dedicated secretaries, for example. The role of typist, administrative assistant, runner, page, proofreader, and data entry specialist are now largely automated, or are quickly being automated. This automation never completely replaces the human’s role in an organization, but it does shift the work from one role to the other. Instead of having a typist/secretary for example, along with a pool of pages and assistants, an executive might now have one assistant. Or there might be a secretary shared by several managers. Or in many cases, the managers themselves do the roles formerly assigned to underlings.
At the highest levels, jobs in management of people remain relatively safe from automation, but the responsibilities of those jobs may increase, as their ability to leverage data and produce important management decisions also increases. There may be fewer managers, who have more overall responsibility than before. This doesn’t always happen at the highest levels of large corporations, but smaller companies definitely expect their employees to “do more with less.” The days of being a career assistant for a major corporation are now largely gone. Only the most skilled assistants have a role left, and compete against far stronger candidates for those roles.
How to Plan for Automation in Your Career
The good news, perhaps, is that despite our fear of automation “taking our jobs,” the truth is that the job market and education have been shifting for decades in anticipation of these changes. Very few people today attend “secretary school” for example, or enter page programs (essentially professional career assistants) at major corporations or in government. Many of these roles are now seen not as professional careers, but as starting points for a different kind of career.
There are of course career-making jobs in all the same industries we’ve mentioned, but they will be both fewer and more demanding. Jobs close to automated technologies require more experience with technology, more knowledge of data science, and a better understanding of the underlying business processes taking place. A secretary, data entry specialist, or assistant to the assistant director role in the 1960s required almost no internal knowledge of a company or business process to get started. Today, these roles go to people with intern experience, and often to people who have an underlying business education, such as an MBA, or a business or economics degree.
While this does mean that the fewer roles for this kind of worker are more competitive, there are also fewer people competing for those roles, which does have other benefits to society and workplaces. Today, gender, sexuality and race tolerance and acceptance have improved in the workplace, and no doubt this is connected with the fact that “less responsible” jobs are now competed for by fewer and more qualified applicants, and because companies can no longer afford to hire based on dated societal expectations about the kind of person suited for a particular role.
The downside of this shift should then be obvious as well: there are fewer entry points into large corporations and government work for those of less education or experience. That is its own kind of discrimination and should be addressed as equally damaging as the old kind. Companies and governments must both improve the prospects of those without much formal education, or without the social and economic status that makes getting jobs in the new economy easier.
But that will not be enough. Workers who are susceptible to replacement by machines should prepare themselves by understanding the processes underlying their jobs, and seeking further education when possible, so that they are seen as valuable when and if the exact role they are filling in a corporation is automated, and a company has a decision to make between keeping someone in a slightly evolved role, or laying them off. Unfortunately, even the best workers are liable to find themselves out of a job if they are not ready to pursue new opportunities within a company.
Things That are Least Likely to Be Automated
Then, of course, there are jobs that don’t fit any of the requirements for “easy” automation. Jobs that are complicated to do, even if they are not “skilled,” and that do not involve a lot of routine processes or a lot of available data, are going to continue to be done by people.
By the way, as you may know, the definition of “skilled work” is always biased toward information sector jobs, when in fact many “unskilled” jobs require a great deal of actual skill and talent to do. Try and pick strawberries for a summer, and you’ll learn that there is a great deal of skill involved in the task, no matter what economists or managers may think about it. Other jobs like this are gardening, painting, repair work, carpentry, auto repair, plumbing, food service, food prep, customer care, reception, janitorial work, teaching, and most jobs with a high degree of contact with the public.
Not coincidentally, these often very difficult jobs are commonly underpaid and overworked. However the fact that they are often overworked is a sign that they are extremely difficult to automate. Workers who find themselves with a decreasing set of responsibilities and a job that gets easier over time thanks to computers, is actually a job that is at risk of being automated away. There is a reason that people who have a = lot of contact with the public, and are good at it, are hard to automate away, and hard to hire in the first place. It takes a great deal of skill to do many of these “simple” jobs, if only because they have so many responsibilities relative to how “empowered” they are, and because they are “failure critical” parts of a business: parts that will become immediately apparent if they are not done absolutely correctly.
So if you’re in a job where your presence is absolutely essential, and where your absence would cause an immediate problem to a business: congrats, you’re probably pretty safe from automation.